Portrait Plot: Mean Climate, comparing CMIP5 & CMIP6 models
Generate a static image of Portrait plot for PMP mean climate metrics obtained from CMIP5 and CMIP6 models, and compare multi-model averaged statistics from each group.
Written by Jiwoo Lee (LLNL/PCMDI)
Last update: 2022. 10
1. Read data from JSON files
Input data for portrait plot is expected as a set a (stacked or list of) 2-d numpy array(s) with list of strings for x and y axes labels.
1.1 Download PMP output JSON files for CMIP models
[1]:
import glob
import os
import numpy as np
import requests
import pandas as pd
from pcmdi_metrics.graphics import download_archived_results
PMP output files downloadable from the PMP results archive.
[2]:
vars = ['pr', 'prw', 'psl', 'rlds', 'rltcre', 'rlus', 'rlut', 'rlutcs', 'rsds', 'rsdscs', 'rsdt', 'rstcre', 'rsut', 'rsutcs', 'sfcWind',
'ta-200', 'ta-850', 'tas', 'tauu', 'ts', 'ua-200', 'ua-850', 'va-200', 'va-850', 'zg-500']
[3]:
json_dir = './json_files'
[4]:
mip = "cmip5"
exp = "historical"
data_version = "v20220928"
[5]:
for var in vars:
path = "metrics_results/mean_climate/"+mip+"/"+exp+"/"+data_version+"/"+var+"."+mip+"."+exp+".regrid2.2p5x2p5."+data_version+".json"
download_archived_results(path, json_dir)
[6]:
mip = "cmip6"
exp = "historical"
data_version = "v20220928"
[7]:
for var in vars:
path = "metrics_results/mean_climate/"+mip+"/"+exp+"/"+data_version+"/"+var+"."+mip+"."+exp+".regrid2.2p5x2p5."+data_version+".json"
download_archived_results(path, json_dir)
Check JSON files
[8]:
json_list_1 = sorted(glob.glob(os.path.join(json_dir, '*.cmip5.' + exp + '*' + data_version + '.json')))
json_list_2 = sorted(glob.glob(os.path.join(json_dir, '*.cmip6.' + exp + '*' + data_version + '.json')))
[9]:
print('CMIP5 JSON files:')
for i, json_file in enumerate(json_list_1):
print(i+1, json_file.split('/')[-1])
print('CMIP6 JSON files:')
for i, json_file in enumerate(json_list_2):
print(i+1, json_file.split('/')[-1])
CMIP5 JSON files:
1 pr.cmip5.historical.regrid2.2p5x2p5.v20220928.json
2 prw.cmip5.historical.regrid2.2p5x2p5.v20220928.json
3 psl.cmip5.historical.regrid2.2p5x2p5.v20220928.json
4 rlds.cmip5.historical.regrid2.2p5x2p5.v20220928.json
5 rltcre.cmip5.historical.regrid2.2p5x2p5.v20220928.json
6 rlus.cmip5.historical.regrid2.2p5x2p5.v20220928.json
7 rlut.cmip5.historical.regrid2.2p5x2p5.v20220928.json
8 rlutcs.cmip5.historical.regrid2.2p5x2p5.v20220928.json
9 rsds.cmip5.historical.regrid2.2p5x2p5.v20220928.json
10 rsdscs.cmip5.historical.regrid2.2p5x2p5.v20220928.json
11 rsdt.cmip5.historical.regrid2.2p5x2p5.v20220928.json
12 rstcre.cmip5.historical.regrid2.2p5x2p5.v20220928.json
13 rsut.cmip5.historical.regrid2.2p5x2p5.v20220928.json
14 rsutcs.cmip5.historical.regrid2.2p5x2p5.v20220928.json
15 sfcWind.cmip5.historical.regrid2.2p5x2p5.v20220928.json
16 ta-200.cmip5.historical.regrid2.2p5x2p5.v20220928.json
17 ta-850.cmip5.historical.regrid2.2p5x2p5.v20220928.json
18 tas.cmip5.historical.regrid2.2p5x2p5.v20220928.json
19 tauu.cmip5.historical.regrid2.2p5x2p5.v20220928.json
20 ts.cmip5.historical.regrid2.2p5x2p5.v20220928.json
21 ua-200.cmip5.historical.regrid2.2p5x2p5.v20220928.json
22 ua-850.cmip5.historical.regrid2.2p5x2p5.v20220928.json
23 va-200.cmip5.historical.regrid2.2p5x2p5.v20220928.json
24 va-850.cmip5.historical.regrid2.2p5x2p5.v20220928.json
25 zg-500.cmip5.historical.regrid2.2p5x2p5.v20220928.json
CMIP6 JSON files:
1 pr.cmip6.historical.regrid2.2p5x2p5.v20220928.json
2 prw.cmip6.historical.regrid2.2p5x2p5.v20220928.json
3 psl.cmip6.historical.regrid2.2p5x2p5.v20220928.json
4 rlds.cmip6.historical.regrid2.2p5x2p5.v20220928.json
5 rltcre.cmip6.historical.regrid2.2p5x2p5.v20220928.json
6 rlus.cmip6.historical.regrid2.2p5x2p5.v20220928.json
7 rlut.cmip6.historical.regrid2.2p5x2p5.v20220928.json
8 rlutcs.cmip6.historical.regrid2.2p5x2p5.v20220928.json
9 rsds.cmip6.historical.regrid2.2p5x2p5.v20220928.json
10 rsdscs.cmip6.historical.regrid2.2p5x2p5.v20220928.json
11 rsdt.cmip6.historical.regrid2.2p5x2p5.v20220928.json
12 rstcre.cmip6.historical.regrid2.2p5x2p5.v20220928.json
13 rsut.cmip6.historical.regrid2.2p5x2p5.v20220928.json
14 rsutcs.cmip6.historical.regrid2.2p5x2p5.v20220928.json
15 sfcWind.cmip6.historical.regrid2.2p5x2p5.v20220928.json
16 ta-200.cmip6.historical.regrid2.2p5x2p5.v20220928.json
17 ta-850.cmip6.historical.regrid2.2p5x2p5.v20220928.json
18 tas.cmip6.historical.regrid2.2p5x2p5.v20220928.json
19 tauu.cmip6.historical.regrid2.2p5x2p5.v20220928.json
20 ts.cmip6.historical.regrid2.2p5x2p5.v20220928.json
21 ua-200.cmip6.historical.regrid2.2p5x2p5.v20220928.json
22 ua-850.cmip6.historical.regrid2.2p5x2p5.v20220928.json
23 va-200.cmip6.historical.regrid2.2p5x2p5.v20220928.json
24 va-850.cmip6.historical.regrid2.2p5x2p5.v20220928.json
25 zg-500.cmip6.historical.regrid2.2p5x2p5.v20220928.json
1.2 Extract data from JSON files
Use Metrics
class (that use read_mean_clim_json_files
function underneath) to extract data from the above JSON files.
Parameters
json_list
: list of string, where each element is for path/file for PMP output JSON files
Returned object includes
df_dict
: dictionary that has[stat][season][region]
hierarchy structure storing pandas dataframe for metric numbers (Rows: models, Columns: variables (i.e., 2d array)var_list
: list of string, all variables from JSON filesvar_unit_list
: list of string, all variables and its units from JSON filesvar_ref_dict
: dictonary for reference dataset used for each variableregions
: list of string, regionsstats
: list of string, statistics
[10]:
from pcmdi_metrics.graphics import Metrics
[11]:
library_cmip5 = Metrics(json_list_1, mip="cmip5")
/Users/lee1043/mambaforge/envs/pmp_devel_20240425/lib/python3.10/site-packages/pcmdi_metrics/variability_mode/lib/lib_variability_mode.py:259: UserWarning: pcmdi_metrics.variability_modes.lib.sort_human will be deprecated. Please use pcmdi_metrics.utils.sort_human, instead.
warnings.warn(
Warning: The provided level value 20000 appears to be in Pa. It will be automatically converted to hPa by dividing by 100.
Warning: The provided level value 85000 appears to be in Pa. It will be automatically converted to hPa by dividing by 100.
Warning: The provided level value 20000 appears to be in Pa. It will be automatically converted to hPa by dividing by 100.
Warning: The provided level value 85000 appears to be in Pa. It will be automatically converted to hPa by dividing by 100.
Warning: The provided level value 20000 appears to be in Pa. It will be automatically converted to hPa by dividing by 100.
Warning: The provided level value 85000 appears to be in Pa. It will be automatically converted to hPa by dividing by 100.
Warning: The provided level value 50000 appears to be in Pa. It will be automatically converted to hPa by dividing by 100.
[12]:
library_cmip6 = Metrics(json_list_2, mip="cmip6")
Warning: The provided level value 20000 appears to be in Pa. It will be automatically converted to hPa by dividing by 100.
Warning: The provided level value 85000 appears to be in Pa. It will be automatically converted to hPa by dividing by 100.
Warning: The provided level value 20000 appears to be in Pa. It will be automatically converted to hPa by dividing by 100.
Warning: The provided level value 85000 appears to be in Pa. It will be automatically converted to hPa by dividing by 100.
Warning: The provided level value 20000 appears to be in Pa. It will be automatically converted to hPa by dividing by 100.
Warning: The provided level value 85000 appears to be in Pa. It will be automatically converted to hPa by dividing by 100.
Warning: The provided level value 50000 appears to be in Pa. It will be automatically converted to hPa by dividing by 100.
[13]:
stat = 'rms_xy'
seasons = ['djf', 'mam', 'jja', 'son']
region = 'global'
Select only certain models (optional)
[14]:
selected_models = ['ACCESS', 'BCC', 'CESM', 'Can', 'FGOALS', 'FIO-ESM', 'GFDL', 'IPSL', 'MIROC', 'MPI', 'MRI', 'NorESM']
def selected_models_only(df, selected_models):
# Selected models only
model_names = df['model'].tolist()
for model_name in model_names:
drop_model = True
for keyword in selected_models:
if keyword in model_name:
drop_model = False
break
if drop_model:
df.drop(df.loc[df['model']==model_name].index, inplace=True)
df.reset_index(drop=True, inplace=True)
return df
[15]:
for season in seasons:
# Selected models only
library_cmip5.df_dict[stat][season][region] = selected_models_only(library_cmip5.df_dict[stat][season][region], selected_models)
library_cmip6.df_dict[stat][season][region] = selected_models_only(library_cmip6.df_dict[stat][season][region], selected_models)
Merge data
[16]:
# merge dataframes
combined = library_cmip5.merge(library_cmip6)
/Users/lee1043/mambaforge/envs/pmp_devel_20240425/lib/python3.10/site-packages/pcmdi_metrics/graphics/share/Metrics.py:136: FutureWarning: Downcasting object dtype arrays on .fillna, .ffill, .bfill is deprecated and will change in a future version. Call result.infer_objects(copy=False) instead. To opt-in to the future behavior, set `pd.set_option('future.no_silent_downcasting', True)`
result.df_dict[stat][season][region] = pd.concat(
Add rows of multi-model average statistics for each CMIP group
[17]:
for season in seasons:
# mean value of statistics from multi models in each CMIP
combined.df_dict[stat][season][region].loc['CMIP5 mean'] = library_cmip5.df_dict[stat][season][region].mean(numeric_only=True, skipna=True)
combined.df_dict[stat][season][region].loc['CMIP6 mean'] = library_cmip6.df_dict[stat][season][region].mean(numeric_only=True, skipna=True)
combined.df_dict[stat][season][region].at['CMIP5 mean', 'model'] = 'CMIP5 mean'
combined.df_dict[stat][season][region].at['CMIP6 mean', 'model'] = 'CMIP6 mean'
Customize variables to show
[18]:
var_list = sorted(combined.var_list)
# temporary
var_list.remove('sfcWind')
var_list.remove('ta-850')
var_list.remove('ua-850')
var_list.remove('ua-200')
var_list.remove('va-850')
var_list.remove('va-200')
var_list.remove('tauu')
print('var_list:', var_list)
var_list: ['pr', 'prw', 'psl', 'rlds', 'rltcre', 'rlus', 'rlut', 'rlutcs', 'rsds', 'rsdscs', 'rsdt', 'rstcre', 'rsut', 'rsutcs', 'ta-200', 'tas', 'ts', 'zg-500']
Getting ready for plotting…
[19]:
#combined.df_dict[stat][season][region]
[20]:
landscape = True
data = dict()
for season in seasons:
if landscape:
data[season] = combined.df_dict[stat][season][region][var_list].to_numpy().T
else:
data[season] = combined.df_dict[stat][season][region][var_list].to_numpy()
[21]:
data_all = np.stack([data['djf'], data['mam'], data['jja'], data['son']])
model_names = combined.df_dict[stat][season][region]['model'].tolist()
print('data.shape:', data_all.shape)
print('len(var_list): ', len(var_list))
print('len(model_names): ', len(model_names))
data.shape: (4, 18, 57)
len(var_list): 18
len(model_names): 57
[22]:
if landscape:
yaxis_labels = var_list
xaxis_labels = model_names
else:
xaxis_labels = var_list
yaxis_labels = model_names
1.4 Normalize each column by its median
Use normalize_by_median
function.
Parameters
data
: 2d numpy arrayaxis
: 0 (normalize each column) or 1 (normalize each row), default=0
Return
data_nor
: 2d numpy array
[23]:
if landscape:
axis = 1
figsize = (40, 10)
else:
axis = 0
figsize = (18, 25)
[24]:
from pcmdi_metrics.graphics import normalize_by_median
data_djf_nor = normalize_by_median(data['djf'], axis=axis)
data_mam_nor = normalize_by_median(data['mam'], axis=axis)
data_jja_nor = normalize_by_median(data['jja'], axis=axis)
data_son_nor = normalize_by_median(data['son'], axis=axis)
[25]:
data_all_nor = np.stack([data_djf_nor, data_mam_nor, data_jja_nor, data_son_nor])
data_all_nor.shape
[25]:
(4, 18, 57)
2. Matplotlib-based PMP Visualization Function
[26]:
from pcmdi_metrics.graphics import portrait_plot
Parameters
data
: 2d numpy array, a list of 2d numpy arrays, or a 3d numpy array (i.e. stacked 2d numpy arrays)xaxis_labels
: list of strings, labels for xaixs. Number of list element must consistent to x-axis, or 0 (empty list) to turn off xaxis tick labelsyaxis_labels
: list of strings, labels for yaxis. Number of list element must consistent to y-axis, or 0 (empty list) to turn off yaxis tick labelsfig
:matplotlib.figure
instance to which the portrait plot is plotted. If not provided, use current axes or create a new one. Optional.ax
:matplotlib.axes.Axes
instance to which the portrait plot is plotted. If not provided, use current axes or create a new one. Optional.annotate
: bool, default=False, add annotating text if true, but work only for heatmap style map (i.e., no triangles)annotate_data
: 2d numpy array, default=None. If None, the image’s data is used. Optional.annotate_fontsize
: number (int/float), default=15. Font size for annotationannotate_format
: format for annotate value, default=”{x:.2f}”figsize
: tuple of two numbers (width, height), default=(12, 10), figure size in inchesvrange
: tuple of two numbers, range of value for colorbar. Optional.xaxis_fontsize
: number, default=15, font size for xaxis tick labels. Optional.yaxis_fontsize
: number, default=15, font size for yaxis tick labels. Optional.inner_line_color
: string, default=”k” (black), color for inner lines (triangle edge lines). Optional.inner_line_width
: float, default=0.5, line width for inner lines (triangle edge lines). Optional.cmap
: string, default=”RdBu_r”, name of matplotlib colormap. Optional.cmap_bounds
: list of numbers. If given, discrete colors are applied. Optional.cbar_label
: string, default=None, label for colorbar. Optional.cbar_label_fontsize
: number, default=15, font size for colorbar labels. Optional.cbar_tick_fontsize
: number, default=12, font size for colorbar tick labels. Optional.cbar_kw
: A dictionary with arguments tomatplotlib.Figure.colorbar
. Optional.colorbar_off
: Trun off colorbar if True. Optional.missing_color
: color, default=”grey”,matplotlib.axes.Axes.set_facecolor
parameter. Optional.invert_yaxis
: bool, default=True, place y=0 at top on the plot. Optional.box_as_square
: bool, default=False, make each box as square. Optional.legend_on
: bool, default=False, show legend (only for 2 or 4 triangles portrait plot). Optional.legend_labels
: list of strings, legend labels for triangls. Optional.legend_box_xy
: tuple of numbers, position of legend box’s upper-left corner. Optional. (lower-left ifinvert_yaxis=False
), inaxes
coordinate. Optional.legend_box_size
: number, size of legend box. Optional.legend_lw
: number, line width of legend, default=1. Optional.legend_fontsize
: number, font size for legend, default=14. Optional.logo_rect
: sequence of float. The dimensions [left, bottom, width, height] of the the PMP logo. Optional. All quantities are in fractions of figure width and height. Optionallogo_off
: bool, default=False, turn off PMP logo. Optional.debug
: bool, default=False, if true print more message when running that help debugging. Optional.
Return
fig
: matplotlib component for figureax
: matplotlib component for axiscbar
: matplotlib component for colorbar (not returned if colorbar_off=True)
3. Plot
3.1 Portrait Plot with 4 Triangles (4 seasons)
data order is clockwise from top: top, right, bottom, left
[27]:
fig, ax, cbar = portrait_plot(data_all_nor,
xaxis_labels=xaxis_labels,
yaxis_labels=yaxis_labels,
cbar_label='RMSE, normalized by median',
box_as_square=True,
vrange=(-0.5, 0.5),
figsize=figsize,
xaxis_fontsize=13,
cmap='RdYlBu_r',
cmap_bounds=[-0.5, -0.4, -0.3, -0.2, -0.1, 0, 0.1, 0.2, 0.3, 0.4, 0.5],
cbar_kw={"extend": "both"},
missing_color='grey',
legend_on=True,
legend_labels=['DJF', 'MAM', 'JJA', 'SON'],
#legend_box_xy=(1.25, 1),
legend_box_xy=(1.1, 1.2),
legend_box_size=5,
legend_lw=1,
legend_fontsize=12.5,
#logo_rect = [0.85, 0.15, 0.07, 0.07],
logo_off=True,
)
ax.set_xticklabels(xaxis_labels, rotation=45, va='bottom', ha="left")
# Add title
ax.set_title("Seasonal climatology Model Performance (global)", fontsize=30, pad=30)
# Add Watermark
ax.text(0.5, 0.5, 'Example', transform=ax.transAxes,
fontsize=100, color='black', alpha=0.6,
ha='center', va='center', rotation=25)
[27]:
Text(0.5, 0.5, 'Example')
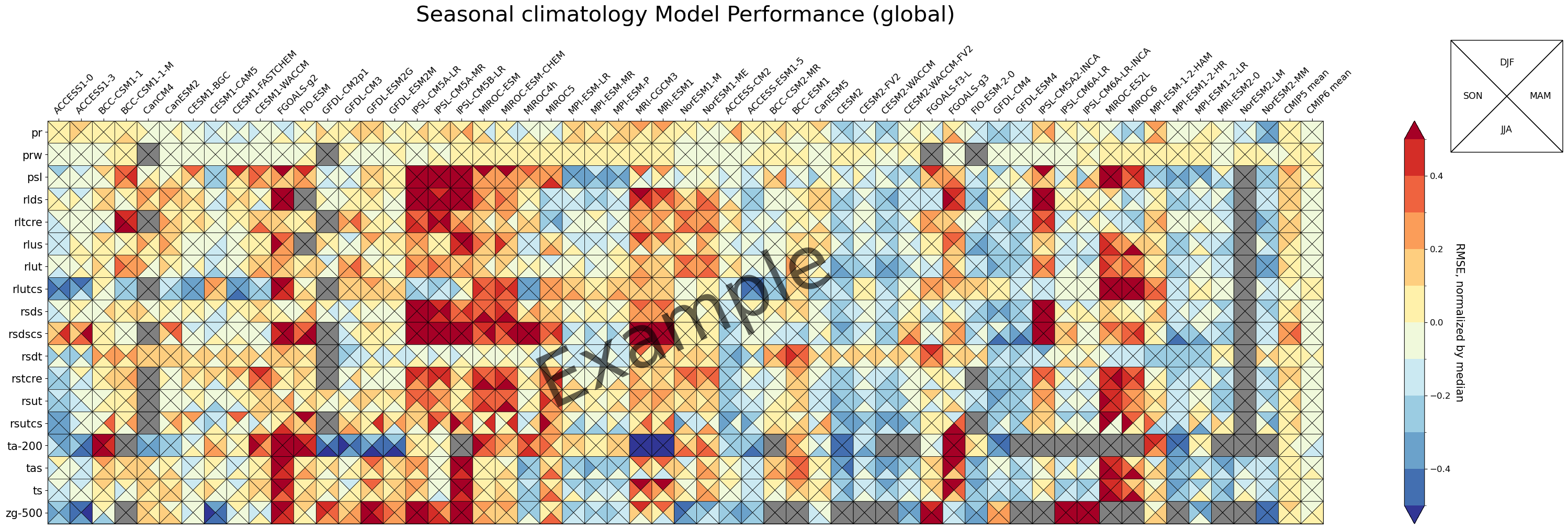
[28]:
# Save figure as an image file
fig.savefig('mean_clim_portrait_plot_4seasons_cmip56.png', facecolor='w', bbox_inches='tight')