Parallel Coordinate Plot: Mean Climate
Generate a static image of Parallel coordinate plot using PMP, for mean climate metrics.
Author: Jiwoo Lee (2021-07)
Last update: 2024-03
1. Read data from JSON files
Input data for parallel coordinate plot is expected as a set a (stacked or list of) 2-d numpy array(s) with list of strings for x and y axes labels.
1.1 Provide PMP output JSON files
[1]:
import glob
import os
import numpy as np
import requests
PMP output files downloadable from the PMP results archive.
[2]:
vars = ['pr', 'prw', 'psl', 'rlds', 'rltcre', 'rlus', 'rlut', 'rlutcs', 'rsds', 'rsdscs', 'rsdt', 'rstcre', 'rsut', 'rsutcs', 'sfcWind',
'ta-200', 'ta-850', 'tas', 'tauu', 'ts', 'ua-200', 'ua-850', 'va-200', 'va-850', 'zg-500']
mip = "cmip6"
exp = "historical"
data_version = "v20230823"
json_dir = './json_files'
os.makedirs(json_dir, exist_ok=True)
for var in vars:
url = "https://raw.githubusercontent.com/PCMDI/pcmdi_metrics_results_archive/main/" + \
"metrics_results/mean_climate/"+mip+"/"+exp+"/"+data_version+"/"+var+"."+mip+"."+exp+".regrid2.2p5x2p5."+data_version+".json"
r = requests.get(url, allow_redirects=True)
filename = os.path.join(json_dir, url.split('/')[-1])
with open(filename, 'wb') as file:
file.write(r.content)
print('Download completed:', filename)
Download completed: ./json_files/pr.cmip6.historical.regrid2.2p5x2p5.v20230823.json
Download completed: ./json_files/prw.cmip6.historical.regrid2.2p5x2p5.v20230823.json
Download completed: ./json_files/psl.cmip6.historical.regrid2.2p5x2p5.v20230823.json
Download completed: ./json_files/rlds.cmip6.historical.regrid2.2p5x2p5.v20230823.json
Download completed: ./json_files/rltcre.cmip6.historical.regrid2.2p5x2p5.v20230823.json
Download completed: ./json_files/rlus.cmip6.historical.regrid2.2p5x2p5.v20230823.json
Download completed: ./json_files/rlut.cmip6.historical.regrid2.2p5x2p5.v20230823.json
Download completed: ./json_files/rlutcs.cmip6.historical.regrid2.2p5x2p5.v20230823.json
Download completed: ./json_files/rsds.cmip6.historical.regrid2.2p5x2p5.v20230823.json
Download completed: ./json_files/rsdscs.cmip6.historical.regrid2.2p5x2p5.v20230823.json
Download completed: ./json_files/rsdt.cmip6.historical.regrid2.2p5x2p5.v20230823.json
Download completed: ./json_files/rstcre.cmip6.historical.regrid2.2p5x2p5.v20230823.json
Download completed: ./json_files/rsut.cmip6.historical.regrid2.2p5x2p5.v20230823.json
Download completed: ./json_files/rsutcs.cmip6.historical.regrid2.2p5x2p5.v20230823.json
Download completed: ./json_files/sfcWind.cmip6.historical.regrid2.2p5x2p5.v20230823.json
Download completed: ./json_files/ta-200.cmip6.historical.regrid2.2p5x2p5.v20230823.json
Download completed: ./json_files/ta-850.cmip6.historical.regrid2.2p5x2p5.v20230823.json
Download completed: ./json_files/tas.cmip6.historical.regrid2.2p5x2p5.v20230823.json
Download completed: ./json_files/tauu.cmip6.historical.regrid2.2p5x2p5.v20230823.json
Download completed: ./json_files/ts.cmip6.historical.regrid2.2p5x2p5.v20230823.json
Download completed: ./json_files/ua-200.cmip6.historical.regrid2.2p5x2p5.v20230823.json
Download completed: ./json_files/ua-850.cmip6.historical.regrid2.2p5x2p5.v20230823.json
Download completed: ./json_files/va-200.cmip6.historical.regrid2.2p5x2p5.v20230823.json
Download completed: ./json_files/va-850.cmip6.historical.regrid2.2p5x2p5.v20230823.json
Download completed: ./json_files/zg-500.cmip6.historical.regrid2.2p5x2p5.v20230823.json
Uncompress PMP output archive file
Check JSON files
[3]:
json_list = sorted(glob.glob(os.path.join(json_dir, '*' + mip + '*' + data_version + '.json')))
for json_file in json_list:
print(json_file.split('/')[-1])
pr.cmip6.historical.regrid2.2p5x2p5.v20230823.json
prw.cmip6.historical.regrid2.2p5x2p5.v20230823.json
psl.cmip6.historical.regrid2.2p5x2p5.v20230823.json
rlds.cmip6.historical.regrid2.2p5x2p5.v20230823.json
rltcre.cmip6.historical.regrid2.2p5x2p5.v20230823.json
rlus.cmip6.historical.regrid2.2p5x2p5.v20230823.json
rlut.cmip6.historical.regrid2.2p5x2p5.v20230823.json
rlutcs.cmip6.historical.regrid2.2p5x2p5.v20230823.json
rsds.cmip6.historical.regrid2.2p5x2p5.v20230823.json
rsdscs.cmip6.historical.regrid2.2p5x2p5.v20230823.json
rsdt.cmip6.historical.regrid2.2p5x2p5.v20230823.json
rstcre.cmip6.historical.regrid2.2p5x2p5.v20230823.json
rsut.cmip6.historical.regrid2.2p5x2p5.v20230823.json
rsutcs.cmip6.historical.regrid2.2p5x2p5.v20230823.json
sfcWind.cmip6.historical.regrid2.2p5x2p5.v20230823.json
ta-200.cmip6.historical.regrid2.2p5x2p5.v20230823.json
ta-850.cmip6.historical.regrid2.2p5x2p5.v20230823.json
tas.cmip6.historical.regrid2.2p5x2p5.v20230823.json
tauu.cmip6.historical.regrid2.2p5x2p5.v20230823.json
ts.cmip6.historical.regrid2.2p5x2p5.v20230823.json
ua-200.cmip6.historical.regrid2.2p5x2p5.v20230823.json
ua-850.cmip6.historical.regrid2.2p5x2p5.v20230823.json
va-200.cmip6.historical.regrid2.2p5x2p5.v20230823.json
va-850.cmip6.historical.regrid2.2p5x2p5.v20230823.json
zg-500.cmip6.historical.regrid2.2p5x2p5.v20230823.json
1.2 Extract data from JSON files
Use Metrics
class (that use read_mean_clim_json_files
function underneath) to extract data from the above JSON files.
Parameters
json_list
: list of string, where each element is for path/file for PMP output JSON files
Returned object includes
df_dict
: dictionary that has[stat][season][region]
hierarchy structure storing pandas dataframe for metric numbers (Rows: models, Columns: variables (i.e., 2d array)var_list
: list of string, all variables from JSON filesvar_unit_list
: list of string, all variables and its units from JSON filesvar_ref_dict
: dictonary for reference dataset used for each variableregions
: list of string, regionsstats
: list of string, statistics
[4]:
from pcmdi_metrics.graphics import Metrics
[5]:
library = Metrics(json_list)
[6]:
df_dict = library.df_dict
var_list = library.var_list
var_unit_list = library.var_unit_list
regions = library.regions
stats = library.stats
[7]:
print('var_list:', var_list)
print('var_unit_list:', var_unit_list)
print("len(var_list:", len(var_list))
print('regions:', regions)
print('stats:', stats)
var_list: ['pr', 'prw', 'psl', 'rlds', 'rltcre', 'rlus', 'rlut', 'rlutcs', 'rsds', 'rsdscs', 'rsdt', 'rstcre', 'rsut', 'rsutcs', 'sfcWind', 'ta-200', 'ta-850', 'tas', 'tauu', 'ts', 'ua-200', 'ua-850', 'va-200', 'va-850', 'zg-500']
var_unit_list: ['pr [kg m-2 s-1]', 'prw [kg m-2]', 'psl [Pa]', 'rlds [W m-2]', 'rltcre [W m-2]', 'rlus [W m-2]', 'rlut [W m-2]', 'rlutcs [W m-2]', 'rsds [W m-2]', 'rsdscs [W m-2]', 'rsdt [W m-2]', 'rstcre [W m-2]', 'rsut [W m-2]', 'rsutcs [W m-2]', 'sfcWind [m s-1]', 'ta-200 [K]', 'ta-850 [K]', 'tas [K]', 'tauu [Pa]', 'ts [K]', 'ua-200 [m s-1]', 'ua-850 [m s-1]', 'va-200 [m s-1]', 'va-850 [m s-1]', 'zg-500 [m]']
len(var_list: 25
regions: ['SHEX_ocean', 'NHEX_land', 'ocean', 'NHEX', 'TROPICS', 'ocean_SHEX', 'land_NHEX', 'SHEX', 'SHEX_land', 'ocean_50S50N', 'global', 'land', 'NHEX_ocean']
stats: ['bias_xy', 'cor_xy', 'mae_xy', 'mean-obs_xy', 'mean_xy', 'rms_devzm', 'rms_xy', 'rms_xyt', 'rms_y', 'rmsc_xy', 'std-obs_xy', 'std-obs_xy_devzm', 'std-obs_xyt', 'std_xy', 'std_xy_devzm', 'std_xyt']
[8]:
df_dict['rms_xyt']['ann']['global']
[8]:
model | run | model_run | pr | prw | psl | rlds | rltcre | rlus | rlut | ... | ta-200 | ta-850 | tas | tauu | ts | ua-200 | ua-850 | va-200 | va-850 | zg-500 | |
---|---|---|---|---|---|---|---|---|---|---|---|---|---|---|---|---|---|---|---|---|---|
0 | ACCESS-CM2 | r1i1p1f1 | ACCESS-CM2_r1i1p1f1 | 1.949 | 126.951 | 267.209 | 13.464 | 9.509 | 10.893 | 12.794 | ... | 2.803 | 1.616 | 2.237 | 0.038 | 2.368 | 4.969 | 1.557 | 2.097 | 0.961 | 26.936 |
1 | ACCESS-ESM1-5 | r1i1p1f1 | ACCESS-ESM1-5_r1i1p1f1 | 1.911 | 127.011 | 263.105 | 10.953 | 8.150 | 10.449 | 12.022 | ... | 2.383 | 1.294 | 1.931 | 0.035 | 2.049 | 4.467 | 1.624 | 2.151 | 0.989 | 27.823 |
2 | AWI-CM-1-1-MR | r1i1p1f1 | AWI-CM-1-1-MR_r1i1p1f1 | 1.758 | 127.072 | 223.345 | 11.130 | 8.484 | 8.574 | 9.879 | ... | 2.048 | 1.182 | 1.425 | 0.029 | 1.567 | 3.282 | 1.422 | 1.986 | 0.888 | 21.041 |
3 | AWI-ESM-1-1-LR | r1i1p1f1 | AWI-ESM-1-1-LR_r1i1p1f1 | 2.021 | 127.125 | 249.276 | 14.488 | 9.693 | 12.189 | 12.794 | ... | 3.767 | 2.116 | 1.915 | 0.034 | 2.220 | 4.433 | 1.825 | 2.295 | 1.061 | NaN |
4 | BCC-CSM2-MR | r1i1p1f1 | BCC-CSM2-MR_r1i1p1f1 | 1.863 | 127.155 | 316.509 | 13.636 | 8.158 | 11.373 | 11.124 | ... | 2.457 | 1.818 | 2.520 | 0.037 | 2.340 | 4.853 | 1.974 | 2.215 | 1.146 | 30.647 |
... | ... | ... | ... | ... | ... | ... | ... | ... | ... | ... | ... | ... | ... | ... | ... | ... | ... | ... | ... | ... | ... |
60 | NorESM2-MM | r1i1p1f1 | NorESM2-MM_r1i1p1f1 | 1.204 | 127.056 | 223.097 | 11.446 | 6.701 | 9.952 | 8.217 | ... | NaN | NaN | 1.759 | 0.030 | 1.967 | 3.538 | 1.467 | NaN | 0.931 | 21.945 |
61 | SAM0-UNICON | r1i1p1f1 | SAM0-UNICON_r1i1p1f1 | 1.612 | 126.982 | 226.741 | 13.173 | 9.219 | 10.983 | 11.228 | ... | 3.753 | 1.444 | 2.368 | 0.035 | 2.512 | 3.689 | 1.729 | 2.170 | 0.952 | 24.147 |
62 | TaiESM1 | r1i1p1f1 | TaiESM1_r1i1p1f1 | 1.562 | 126.969 | 233.954 | 11.386 | 8.617 | 9.574 | 9.703 | ... | NaN | NaN | 2.027 | 0.040 | 2.207 | NaN | 1.618 | NaN | 0.895 | NaN |
63 | UKESM1-0-LL | r1i1p1f2 | UKESM1-0-LL_r1i1p1f2 | 1.749 | 126.845 | 256.131 | 13.240 | 8.769 | 10.714 | 10.896 | ... | NaN | NaN | 2.237 | 0.033 | 2.346 | 3.757 | 1.404 | 1.905 | 0.882 | NaN |
64 | UKESM1-1-LL | r1i1p1f2 | UKESM1-1-LL_r1i1p1f2 | 1.770 | 126.928 | 256.194 | 10.833 | 8.865 | 9.740 | 10.636 | ... | 2.333 | 1.145 | 1.766 | 0.033 | 2.064 | 3.909 | 1.407 | 1.920 | 0.886 | NaN |
65 rows × 28 columns
[9]:
# Simple re-order variables
if 'zg-500' in var_list and 'sfcWind' in var_list:
var_list.remove('zg-500')
idx_sfcWind = var_list.index('sfcWind')
var_list.insert(idx_sfcWind+1, 'zg-500')
if 'ta-850' in var_list:
var_list.remove('ta-850')
print("var_list:", var_list)
print("len(var_list:", len(var_list))
var_list: ['pr', 'prw', 'psl', 'rlds', 'rltcre', 'rlus', 'rlut', 'rlutcs', 'rsds', 'rsdscs', 'rsdt', 'rstcre', 'rsut', 'rsutcs', 'sfcWind', 'zg-500', 'ta-200', 'tas', 'tauu', 'ts', 'ua-200', 'ua-850', 'va-200', 'va-850']
len(var_list: 24
[10]:
data = df_dict['rms_xyt']['ann']['global'][var_list].to_numpy()
model_names = df_dict['rms_xyt']['ann']['global']['model'].tolist()
#metric_names = ['\n['.join(var_unit.split(' [')) for var_unit in var_unit_list]
metric_names = var_list
models_to_highlight = ['E3SM-1-0', 'E3SM-1-1', 'E3SM-1-1-ECA', 'E3SM-2-0']
print('data.shape:', data.shape)
print('len(metric_names): ', len(metric_names))
print('len(model_names): ', len(model_names))
data.shape: (65, 24)
len(metric_names): 24
len(model_names): 65
[11]:
units_all = 'prw [kg m-2], pr [mm d-1], psl [Pa], rlds [W m-2], rsdscs [W m-2], rltcre [W m-2], rlus [W m-2], rlut [W m-2], rlutcs [W m-2], rsds [W m-2], rsdt [W m-2], rstcre [W m-2], rsus [W m-2], rsut [W m-2], rsutcs [W m-2], sfcWind [m s-1], zg-500 [m], ta-200 [K], ta-850 [K], tas [K], ts [K], ua-200 [m s-1], ua-850 [m s-1], uas [m s-1], va-200 [m s-1], va-850 [m s-1], vas [m s-1], tauu [Pa]'
units_all.split(', ')
var_unit_list = []
for var in var_list:
found = False
for var_units in units_all.split(', '):
tmp1 = var_units.split(' [')[0]
#print(var, tmp1)
if tmp1 == var:
unit = '[' + var_units.split(' [')[1]
var_unit_list.append(var + '\n' + unit)
found = True
break
if found is False:
print(var, 'not found')
print('var_unit_list:', var_unit_list)
metric_names = var_unit_list
var_unit_list: ['pr\n[mm d-1]', 'prw\n[kg m-2]', 'psl\n[Pa]', 'rlds\n[W m-2]', 'rltcre\n[W m-2]', 'rlus\n[W m-2]', 'rlut\n[W m-2]', 'rlutcs\n[W m-2]', 'rsds\n[W m-2]', 'rsdscs\n[W m-2]', 'rsdt\n[W m-2]', 'rstcre\n[W m-2]', 'rsut\n[W m-2]', 'rsutcs\n[W m-2]', 'sfcWind\n[m s-1]', 'zg-500\n[m]', 'ta-200\n[K]', 'tas\n[K]', 'tauu\n[Pa]', 'ts\n[K]', 'ua-200\n[m s-1]', 'ua-850\n[m s-1]', 'va-200\n[m s-1]', 'va-850\n[m s-1]']
[12]:
df_dict['rms_xyt']['ann']['global'][var_list].columns
[12]:
Index(['pr', 'prw', 'psl', 'rlds', 'rltcre', 'rlus', 'rlut', 'rlutcs', 'rsds',
'rsdscs', 'rsdt', 'rstcre', 'rsut', 'rsutcs', 'sfcWind', 'zg-500',
'ta-200', 'tas', 'tauu', 'ts', 'ua-200', 'ua-850', 'va-200', 'va-850'],
dtype='object')
2. Plot
[13]:
from pcmdi_metrics.graphics import parallel_coordinate_plot
Parameters
data
: 2-d numpy array for metricsmetric_names
: list, names of metrics for individual vertical axes (axis=1)model_names
: list, name of models for markers/lines (axis=0)models_to_highlight
: list, default=None, List of models to highlight as lines or markermodels_to_highlight_by_line
: bool, default=True, highlight as lines. If False, as markermodels_to_highlight_colors
: list, default=None, List of colors for models to highlight as linesmodels_to_highlight_labels
: list, default=None, List of string labels for models to highlight as linesmodels_to_highlight_markers
: list, matplotlib markers for models to highlight if as markermodels_to_highlight_markers_size
: float, size of matplotlib markers for models to highlight if as markerfig
:matplotlib.figure
instance to which the parallel coordinate plot is plotted. If not provided, use current axes or create a new one. Optional.ax
:matplotlib.axes.Axes
instance to which the parallel coordinate plot is plotted. If not provided, use current axes or create a new one. Optional.figsize
: tuple (two numbers), default=(15,5), image sizeshow_boxplot
: bool, default=False, show box and wiskers plotshow_violin
: bool, default=False, show violin plotviolin_colors
: tuple or list containing two strings for colors of violin. Default=(“lightgrey”, “pink”)violin_label
: string to label the violin plot, when violin plot is not splited. Default is None.title
: string, default=None, plot titleidentify_all_models
: bool, default=True. Show and identify all models using markersxtick_labelsize
: number, fontsize for x-axis tick labels (optional)ytick_labelsize
: number, fontsize for x-axis tick labels (optional)colormap
: string, default=’viridis’, matplotlib colormapnum_color
: integer, default=20, how many color to use.legend_off
: bool, default=False, turn off legendlegend_ncol
: integer, default=6, number of columns for legend textlegend_bbox_to_anchor
: tuple, defulat=(0.5, -0.14), set legend box locationlegend_loc
: string, default=”upper center”, set legend box locationlegend_fontsize
: float, default=8, legend font sizelogo_rect
: sequence of float. The dimensions [left, bottom, width, height] of the new Axes. All quantities are in fractions of figure width and height. Optional.logo_off
: bool, default=False, turn off PMP logomodel_names2
: list of string, should be a subset ofmodel_names
. If given, violin plot will be split into 2 groups. Optional.group1_name
: string, needed for violin plot legend if splited to two groups, for the 1st group. Default is ‘group1’.group2_name
: string, needed for violin plot legend if splited to two groups, for the 2nd group. Default is ‘group2’.comparing_models
: tuple or list containing two strings for models to compare with colors filled between the two lines.fill_between_lines
: bool, default=False, fill color between lines for models in comparing_modelsfill_between_lines_colors
: tuple or list containing two strings of colors for filled between the two lines. Default=(‘red’, ‘green’)arrow_between_lines
: bool, default=False, place arrows between two lines for models in comparing_modelsarrow_between_lines_colors
: tuple or list containing two strings of colors for arrow between the two lines. Default=(‘red’, ‘green’)arrow_alpha
: float, default=1, transparency of arrow (faction between 0 to 1)vertical_center
: string (“median”, “mean”)/float/integer, default=None, adjust range of vertical axis to set center of vertical axis as median, mean, or given numbervertical_center_line
: bool, default=False, show median as linevertical_center_line_label
: str, default=None, label in legend for the horizontal vertical center line. If not given, it will be automatically assigned. It can be turned off by “off”ymax
: int or float, default=None, specify value of vertical axis topymin
: int or float, default=None, specify value of vertical axis bottom
Return
fig
: matplotlib component for figureax
: matplotlib component for axis
[14]:
fig, ax = parallel_coordinate_plot(data, metric_names, model_names, models_to_highlight=models_to_highlight,
title='Mean Climate: RMS_XYT, ANN, Global',
figsize=(21, 7),
colormap='tab20',
xtick_labelsize=10,
logo_rect=[0.8, 0.8, 0.15, 0.15])
fig.text(0.99, -0.45, 'Data version\n'+data_version, transform=ax.transAxes,
fontsize=12, color='black', alpha=0.6, ha='right', va='bottom',)
# Save figure as an image file
fig.savefig('mean_clim_parallel_coordinate_plot_'+data_version+'.png', facecolor='w', bbox_inches='tight')
# Add Watermark
ax.text(0.5, 0.5, 'Example', transform=ax.transAxes,
fontsize=100, color='black', alpha=0.2,
ha='center', va='center', rotation=25)
# Save figure as an image file
fig.savefig('mean_clim_parallel_coordinate_plot_example.png', facecolor='w', bbox_inches='tight')
Passed a quick QC
data.shape: (65, 24)
data.shape: (65, 24)
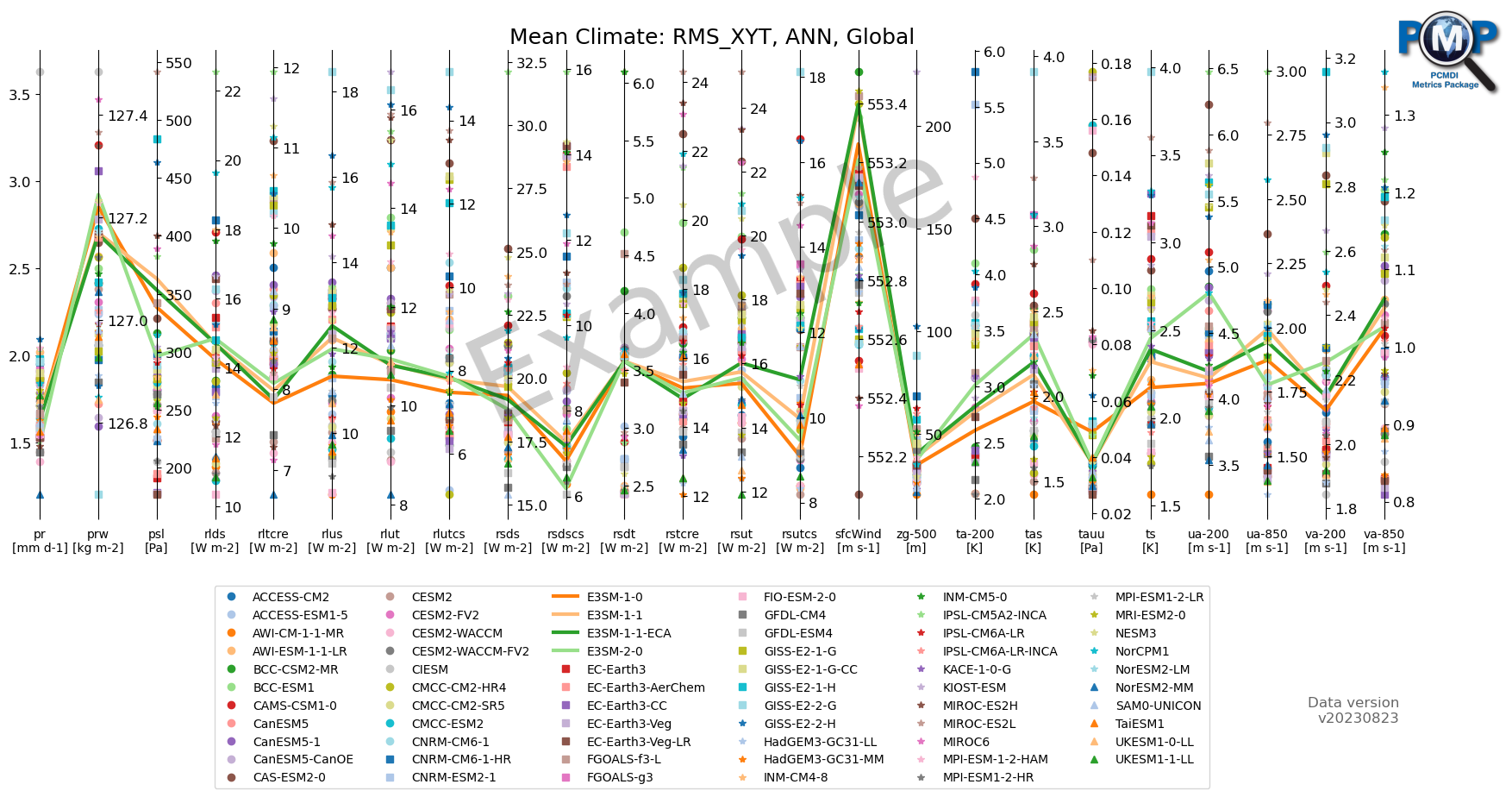